CAMALIOT Project kick-offs successfully achieved
GNSS infrastructure has been growing significantly in recent years. In the space segment, four global constellations are operational, including the European Galileo system. On ground, tens of thousands of permanent GNSS stations are continuously recording data. In addition, millions of Internet-of-things (IoT) devices, including smartphones, use GNSS for positioning. Due to the large number of devices, IoT data offers great potential for GNSS science exploitations, with unprecedented spatio-temporal resolution. However, exploitation of IoT data for GNSS science purposes is currently limited due to multiple challenges.
To address these challenges, CAMALIOT “Application of Machine Learning Technology for GNSS IoT Data Fusion” will integrate IoT and traditional GNSS data sources leveraging on Big Data, Data Fusion and Machine Learning (ML) technologies to unleash innovation opportunities in GNSS science fields. CAMALIOT will extend current capabilities of the GNSS Science Support Centre (GSSC) , with IoT-enabled GNSS data processing pipelines to support several use cases.
Two parallel contracts have been launched to achieve CAMALIOT’s objectives:
- On 18th January 2021, the first kick-off took place with the participation of ESA project team, the main contractor RINA Consulting-Centro Sviluppo Material S.p.A and subcontractors companies Politecnico di Milano, Intelligentia srl, Geomatics Research & Development srl.
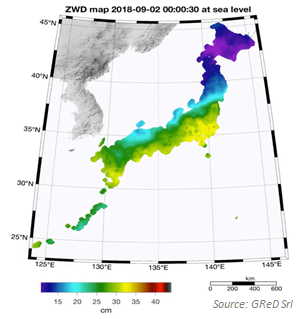
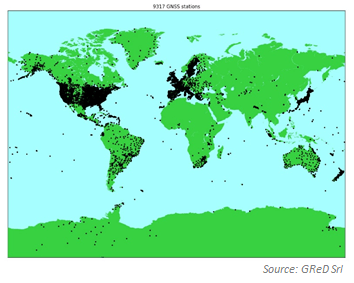
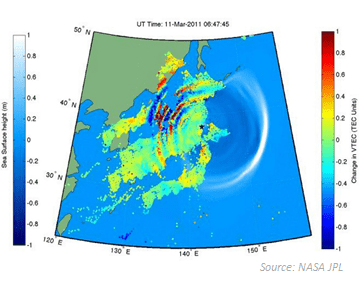
Use Cases
By using an Agile approach, the consortium will develop such software infrastructure as an extension of the current GSSC architecture by focusing on its functional System Domains. The final architecture will extend the current GSSC in the fields of data ingestion, processing and analysis services by implementing the IoT components (including crowdsourcing app) and the ML models and pipeline. The validation of the system domain is conducted through different test cases, mainly focused on the selected GNSS Science use cases.
- On March 2nd 2021, the second kick-off took place with the participation of ESA project team, the main contractor ETH Zurich (ETHZ) and the sub-contractor International Institute for Applied Systems Analysis (IIASA).
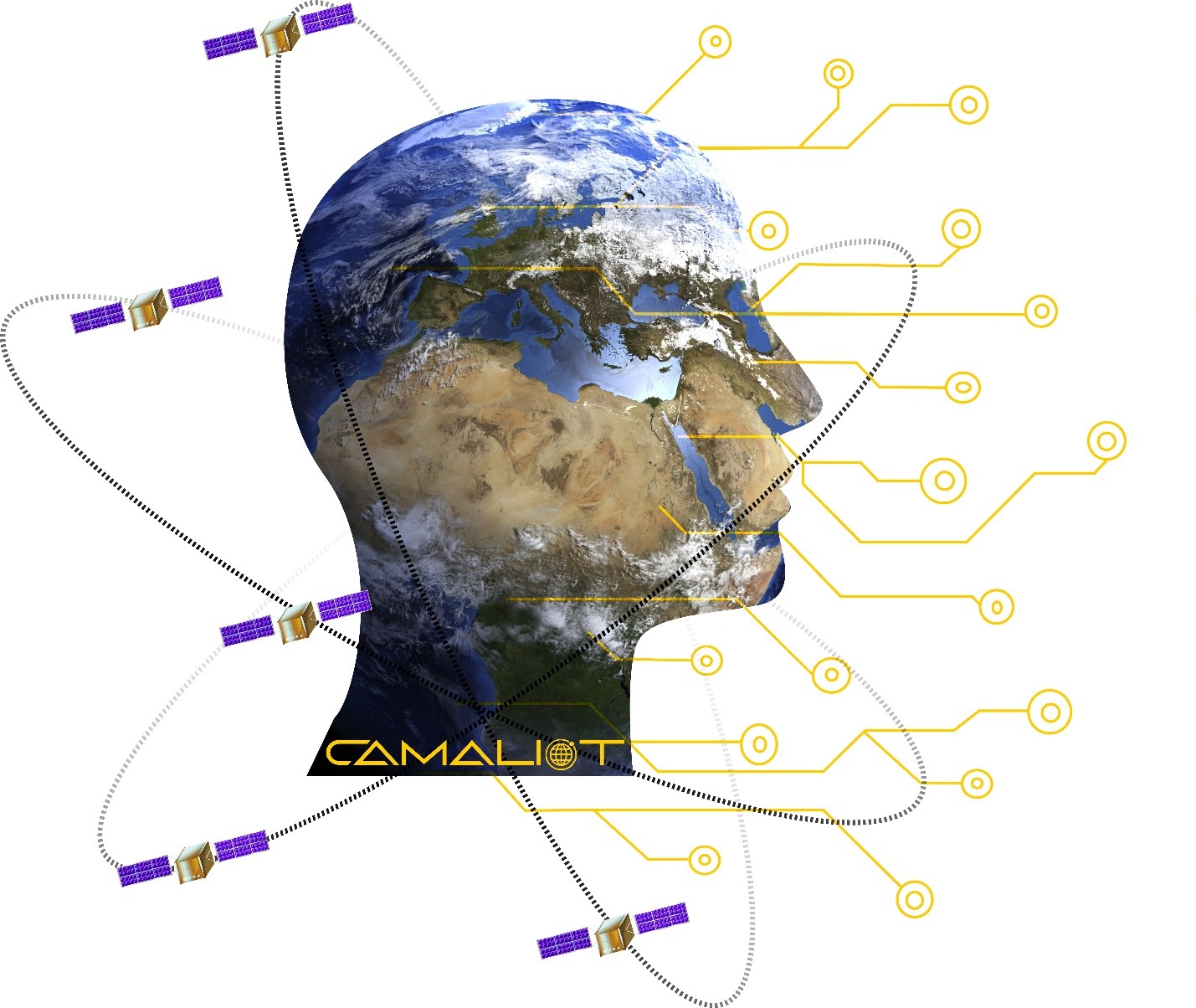
The consortium will develop an Android app for the collection of raw GNSS data from smartphones. A crowdsourcing campaign will be launched, based on the concept of citizen science. In order to cope with the large amounts of GNSS data of heterogeneous quality, new processing methods will be developed that are highly automated and robust. For this purpose, specific ML algorithms will be designed, trained, and applied. Based on the GNSS results, two scientific use cases will be studied, the first focusing on the determination of tropospheric parameters to support weather forecasts on Earth, and the second one concerning the monitoring of space weather, important for satellite operations and communication.